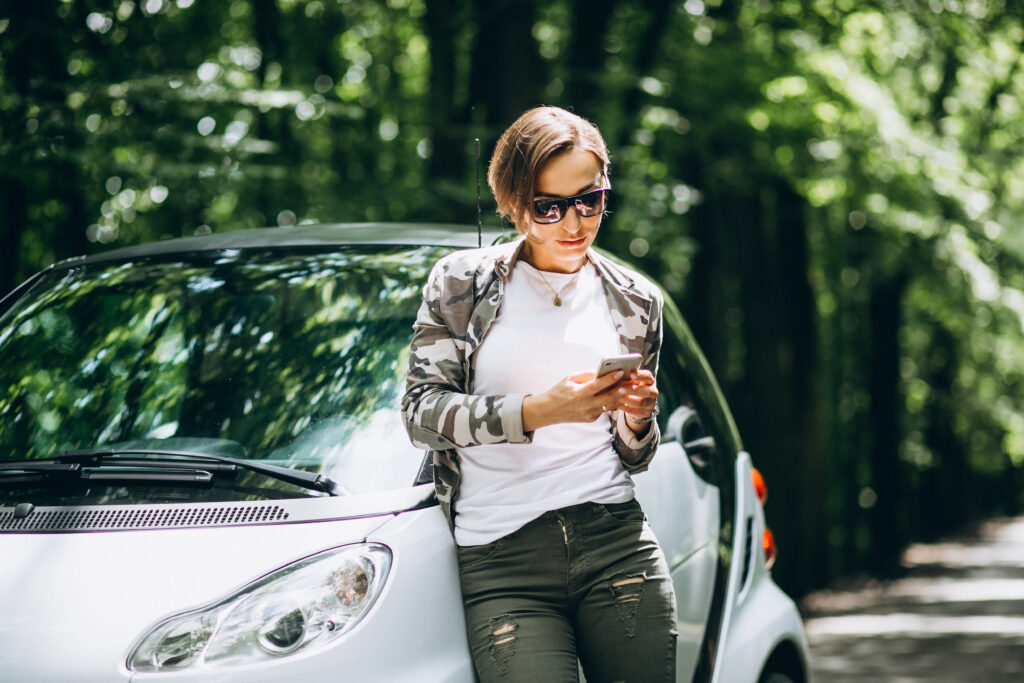
Woman standing by the car in park using phone
AI in Autonomous Vehicles: Navigating the Road Ahead
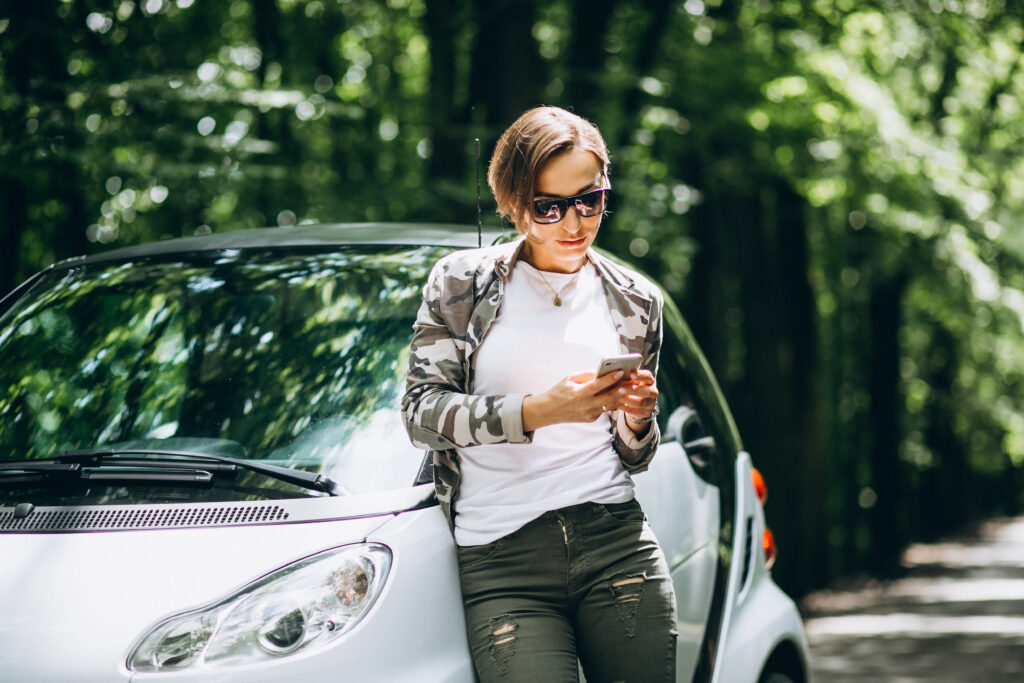
Artificial Intelligence (AI) has become the cornerstone of advancements in autonomous vehicles (AVs), driving innovations in navigation systems, obstacle detection, and decision-making processes. These developments are not only enhancing the safety and efficiency of self-driving cars but are also paving the way for a future where transportation is more intelligent and responsive.
Advancements in Navigation Systems
Modern AVs rely heavily on AI to interpret complex driving environments. By processing data from an array of sensors—including LiDAR, radar, and cameras—AI algorithms construct detailed maps of the vehicle’s surroundings. This sensor fusion enables the vehicle to understand its position relative to other objects and navigate through diverse terrains and traffic conditions. For instance, high-definition (HD) mapping data combined with real-time sensor inputs allow for precise lane-keeping and path planning, ensuring smooth and accurate navigation.
The integration of AI in navigation also facilitates adaptive learning. As AVs encounter various driving scenarios, machine learning algorithms analyze and learn from these experiences, improving the system’s ability to handle similar situations in the future. This continuous learning process enhances the vehicle’s capability to predict and respond to dynamic changes in the environment, such as sudden road closures or unexpected obstacles.
Obstacle detection is a critical component of autonomous driving, and AI has significantly improved the accuracy and reliability of these systems. Through advanced object recognition algorithms, AVs can identify a wide range of obstacles, including pedestrians, cyclists, other vehicles, and stationary objects. By analyzing sensor data in real-time, the AI system classifies these obstacles and assesses potential risks, enabling the vehicle to make informed decisions to avoid collisions.
Moreover, AI-driven navigation systems are becoming increasingly adept at understanding and interpreting traffic signals and road signs. By leveraging computer vision and deep learning techniques, AVs can accurately recognize and react to traffic control devices, ensuring compliance with traffic laws and contributing to overall road safety.
Decision-Making Processes
Decision-making in autonomous vehicles involves determining the optimal driving actions based on the interpreted data from navigation and obstacle detection systems. AI plays a pivotal role in this process by evaluating various driving scenarios and selecting appropriate responses. For instance, when approaching an intersection, the AI system must decide whether to proceed, yield, or stop, considering factors like traffic signals, the presence of other vehicles, and pedestrian activity.
Deep reinforcement learning (DRL) has emerged as a powerful technique in enhancing decision-making capabilities. By training the AI system through simulations, DRL enables the vehicle to learn optimal driving strategies by receiving feedback from its actions. This method allows the system to handle complex and dynamic driving environments, improving its ability to make safe and efficient decisions in real-world scenarios.
Additionally, AI facilitates the development of human-like reasoning in AVs. By incorporating cognitive models that mimic human decision-making processes, AI systems can better understand and predict the behavior of other road users. This capability enhances the vehicle’s interactions with human drivers and pedestrians, promoting safer and more intuitive driving behaviors.
Leading AV Companies
Waymo: Pioneering Autonomous Mobility
Waymo, a subsidiary of Alphabet Inc., has been a trailblazer in the autonomous driving sector. Established initially as Google’s Self-Driving Car Project, Waymo has evolved into a leader in developing fully autonomous vehicles. The company utilizes advanced AI algorithms to process data from LiDAR, radar, and cameras, enabling its vehicles to navigate complex urban environments safely. Recently, Waymo announced plans to expand its testing operations to 10 new cities in 2025, starting with Las Vegas and San Diego, to further refine its self-driving technology.
Tesla: Integrating AI for Enhanced Autopilot
Tesla has been at the forefront of integrating AI into its vehicles, particularly through its Autopilot and Full Self-Driving (FSD) systems. The company employs AI to process data from an array of sensors, including cameras and ultrasonic sensors, to facilitate features such as adaptive cruise control, lane-keeping, and automated parking. Tesla’s AI-driven approach enables its vehicles to navigate complex environments, recognize traffic signals, and make real-time driving decisions, contributing to enhanced safety and efficiency on the road.
Nuro: Revolutionizing Goods Delivery
Nuro focuses on using AI to revolutionize goods delivery through its fleet of autonomous vehicles. Specializing in last-mile delivery, Nuro’s compact, self-driving vehicles are designed to transport goods efficiently and safely without human intervention. The company’s AI systems enable these vehicles to navigate residential areas, recognize obstacles, and make real-time decisions to ensure timely and secure deliveries. Nuro’s innovative approach aims to transform local commerce and logistics by providing a convenient and reliable delivery solution.
Wayve: AI-Driven Learning for Urban Driving
Wayve is pioneering a new era of automated driving by leveraging AI to empower vehicles to perceive, predict, and navigate through dynamic urban environments. The company’s approach focuses on end-to-end deep learning, enabling vehicles to learn from human behavior and adapt to complex driving scenarios. By utilizing AI, Wayve’s technology allows for continuous learning and improvement, facilitating safer and more efficient urban driving experiences.
Motional: Advancing Driverless Technology
Motional is at the forefront of developing fully driverless vehicles that can be seamlessly integrated into mobility networks for autonomous ride-hail and delivery services. The company employs advanced AI to process data from a suite of sensors, enabling its vehicles to navigate complex environments, detect obstacles, and make informed driving decisions. Motional’s commitment to safety and innovation positions it as a key player in the autonomous vehicle industry.
These companies exemplify the transformative impact of AI on autonomous vehicles, each contributing unique innovations that enhance navigation, obstacle detection, and decision-making processes. As AI technology continues to evolve, these advancements are paving the way for a future where autonomous vehicles become a safe and integral part of our transportation ecosystem.
Challenges and Future Directions
Despite significant advancements, the integration of AI in autonomous vehicles presents several challenges. One major concern is the system’s ability to handle edge cases—rare or unforeseen scenarios that were not encountered during training. Ensuring that AI systems can safely manage these situations is critical for widespread adoption.
Ethical considerations also play a crucial role in the deployment of AI-driven AVs. Decisions made by the AI, especially in critical situations, raise questions about accountability and moral judgment. Establishing clear ethical frameworks and regulatory standards is essential to address these concerns and build public trust.
Looking ahead, ongoing research focuses on enhancing the interpretability and transparency of AI systems in AVs. Developing explainable AI models will allow for better understanding and validation of decision-making processes, facilitating improvements in safety and reliability. Additionally, advancements in sensor technology and data processing will continue to refine navigation and obstacle detection capabilities, bringing fully autonomous vehicles closer to reality.
In conclusion, AI is driving transformative changes in the field of autonomous vehicles, with significant progress in navigation, obstacle detection, and decision-making processes. As technology continues to evolve, addressing the associated challenges will be paramount to realizing the full potential of AI-driven transportation systems.
Blog Notes: I was not paid to write this blog post and I will not receive any compensation if you follow the links. I have utilized AI technology and tools in the creation of this blog post but everything has been edited by me for reader consumption and accuracy. If you have any questions please feel free to contact me by completing the contact form on the front page of my website.
Latest Blog Posts
- Sora: OpenAI’s Revolutionary Text-to-Video Generative AI Model
- Open-Source AI for Everyone: DeepSeek’s Role in Bridging the Gap
- From Hedge Fund to AI Powerhouse: The Rise of DeepSeek
- AI Index Report 2024: Key Insights and Highlights
- Transform Your Content Creation: Everything You Need to Know About Pika